Strategies for Effective Data Analysis in Academic Projects
Contents
- 1 Strategies for Effective Data Analysis in Academic Projects
- 1.1 Introduction
- 1.2 Processes in Data analysis of academic projects
- 1.3 Data Collection:
- 1.4 Data Preparation and Cleaning:
- 1.5 Data exploration:
- 1.6 Data modelling:
- 1.7 Data interpretation
- 1.8 How data collection methods affect the type of data analysis
- 1.9 Common tools and methods used in data analysis in academic projects
- 1.10 Conclusion
Introduction
Data analysis is crucial to academic endeavours because it enables academics to extract insights and meaning from large, complex datasets. The primary functions of data analysis in academic initiatives are as follows:
Effective data analysis aids in the development of research questions and hypotheses and identifies the critical factors relevant to the study topic. Researchers can formulate research questions and hypotheses by employing data analysis techniques to identify patterns, trends, and linkages.
Processes in Data analysis of academic projects
-
Data Collection:
When gathering information for academic endeavours, careful thought must be given to data analysis. Researchers can collect data through various methods, such as questionnaires, interviews, and direct observation. However, the quality of the data is determined by the techniques used to collect it. By conducting data analysis, researchers can evaluate the data’s quality and ensure it is reliable and valid.
-
Data Preparation and Cleaning:
The data analysis process includes data cleaning and preparation. This includes resolving mistakes and outliers, removing redundant or missing data, and structuring the information to enable analysis.
-
Data exploration:
This is required to identify data patterns, trends, and linkages. This involves using statistical methods such as regression and correlation analysis to ascertain links between variables.
-
Data modelling:
This is a crucial part of data analysis which involves building models to describe the relationships between variables. This entails using statistical modelling techniques such as logistic regression and linear regression to create predictive models.
-
Data interpretation
The outcomes of data analysis need to be understood in order to make decisions and recommendations. This entails communicating the analysis’s conclusions using visual aids like graphs and charts.
How data collection methods affect the type of data analysis
The methods employed for data gathering significantly impact the types of data analysis that can be performed. The data’s quantity, quality, and structure are influenced by the data-gathering method.
These elements, in turn, influence the appropriate analytical procedures that can be applied. Different data-gathering methods, such as surveys, interviews, observations, and experiments, result in various data requiring a different set of analysis tools.
Data collection methods have a wide range of effects on data analysis. Different methods produce various types of data, including qualitative and quantitative data. Qualitative data provides insightful information on beliefs, attitudes, and actions. It is gathered via multiple techniques, including open-ended surveys and interviews.
In contrast, quantitative data consists of numerical information that can be subjected to statistical analysis. It is usually collected using systematic surveys or experimental measures. When quantitative data is obtained using structured surveys or experiments, statistical analysis techniques like regression analysis, hypothesis testing, and correlation analysis become possible.
Employing these methods can help researchers identify data patterns, correlations, and trends. For example, regression analysis can help understand how independent factors affect a dependent variable, and hypothesis testing can be used to determine the significance of correlations between variables.
Researchers sometimes combine methodologies to collect data using qualitative and quantitative techniques. This approach makes it feasible to do a comprehensive analysis considering both data types, deepening our understanding of the study subject.
Triangulation is a typical process in mixed methods analysis where conclusions are validated by comparing and contrasting findings from many data sources. Whether planning a study or conducting an analysis, it is imperative to carefully examine the most effective data collection strategies based on the research questions and objectives.
When choosing approaches, consider the kind of data required for insightful analysis and the nature of the research challenge. Additionally, ethical considerations and practical constraints may impact the choice of data collection method.
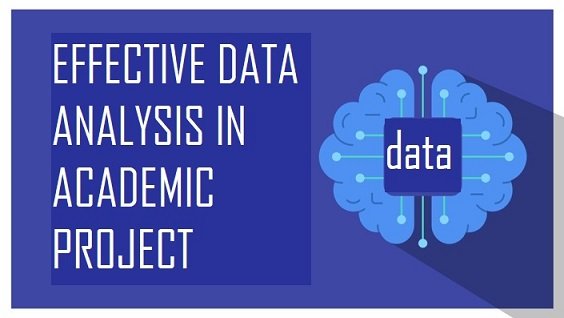
Common tools and methods used in data analysis in academic projects
A key component of academic research is data analysis, and there are several standard tools and techniques for doing so in academic studies. With these instruments and techniques, researchers can extract significant insights from their data, resulting in well-informed findings and important advances in their fields of study.
Packages for Statistical Software In academic data analysis, statistical software programmes like SAS (Statistical Analysis System), R, Stata, and SPSS (Statistical Package for the Social Sciences) are frequently utilised. To help researchers analyse and comprehend their data, these tools include a variety of statistical functions, data manipulation capabilities, and visualisation tools.
Another useful application that’s frequently utilised for data analysis in academic work is Microsoft Excel. Excel is readily available and provides basic statistical analysis, data visualisation, and manipulation, even if it does not have the sophisticated statistical features of specialised statistical applications.
Python’s wealth of libraries, including Pandas, NumPy, and Matplotlib, has made it a popular choice for data analysis programming. Many researchers choose Python because of these modules’ strong data processing, statistical analysis, and visualisation capabilities.
Numerous statistical methods are used in quantitative research to examine numerical data. These methods include regression analysis, factor analysis, descriptive statistics, and inferential statistics (such as t-tests and ANOVA).
Researchers employ these methods to investigate patterns in the data and verify theories. Methods of Qualitative Analysis Analysing non-numerical data to find patterns, themes, and meanings is a key qualitative research component.
Thematic analysis, content analysis, grounded theory, and phenomenological analysis are examples of common methodologies in qualitative analysis. These methods aid researchers in deciphering qualitative data and locating important insights.
Tools for Data Visualisation Data visualization is an integral part of data analysis. Researchers can visualize their data using dashboards, graphs, and charts using tools like Tableau and Power BI.
Conclusion
Academic initiatives must include data analysis since it facilitates the development of insightful findings and decision-making. For analysis to be effective, data quality and integrity must be maintained. To ensure the data is correct and comprehensive, it must be cleaned to remove any mistakes or inconsistencies.
Researchers should also record the steps involved in gathering data and any changes made to the dataset. In addition, choosing the right data analysis tools is crucial for handling and analysing the collected information.
Researchers can utilise statistical tools such as R, Python, SPSS, or Excel depending on how sophisticated the analysis is. With these techniques, researchers can do various studies, such as regression analysis, inferential statistics, and descriptive statistics.
Conducting data analysis requires rigorous analytical approaches pertinent to the research objectives. Researchers should meticulously examine the data in light of the study’s goals and offer lucid justifications for any possible implications.
Academic initiatives require clear communication of the data analysis’s conclusions. Visually representing the results in a way that is easy to read and comprehend may entail making charts, graphs, or tables. Researchers must also write thorough papers or presentations to communicate their findings to their audience effectively.